The Nature of Algorithmic Bias
Algorithmic bias is not inherently malicious; it arises from the design choices, data sets, and assumptions embedded within algorithms that govern social networks. These biases can be subtle yet powerful, shaping what content users see and how they interact with each other. One key aspect of algorithmic bias lies in the way these systems prioritize engagement metrics over accuracy or fairness.
Firstly, algorithms are often designed to maximize user engagement rather than ensure balanced content distribution. This means that posts that generate more likes, shares, and comments tend to be favored, even if they propagate misinformation or reinforce extreme viewpoints. For instance, sensationalist headlines or polarizing opinions can elicit strong emotional responses from users, leading algorithms to promote them excessively, thereby creating echo chambers where diverse perspectives are marginalized.
Secondly, the data used to train these algorithms can inadvertently introduce biases. If historical data is skewed towards certain demographics or political leanings, the resulting models may perpetuate those imbalances. For example, if a social media platform predominantly features content from affluent urban areas while underrepresenting rural communities, users in those regions might feel alienated and less engaged with the broader network. This can further exacerbate existing societal divides, as marginalized groups become less visible or relevant within the algorithmic landscape.
Finally, algorithms often rely on implicit feedback mechanisms that reinforce user behavior. When a user repeatedly engages with certain types of content, the algorithm learns to recommend more similar material in an effort to maintain engagement. Over time, this can create personalized bubbles where users are exposed primarily to information that aligns with their existing beliefs and preferences, further entrenching echo chambers. This feedback loop not only limits exposure to diverse viewpoints but also contributes to the proliferation of misinformation as users become increasingly insulated from challenging or contradictory narratives.
The Impact on User Engagement
The unintentional promotion or suppression of certain content types by algorithms can have profound implications for user engagement, affecting both individual experiences and broader community dynamics. When algorithms prioritize high-engagement content, they often encourage users to post more sensationalist material in order to gain visibility and followers.
Firstly, users may feel incentivized to create emotionally charged posts that are likely to attract attention but do not necessarily contribute positively to the community or foster constructive dialogue. This can lead to a proliferation of inflammatory comments and debates, which, while generating buzz, often fail to build lasting connections or meaningful interactions among users. In some cases, this behavior can result in the alienation of more moderate voices who may be less inclined to engage with provocative content.
Secondly, algorithmic bias can contribute to the formation of echo chambers where users are predominantly exposed to information and opinions that align with their pre-existing beliefs. Within these bubbles, users are less likely to encounter diverse perspectives or challenging ideas, which can limit intellectual growth and critical thinking. Over time, this lack of exposure to alternative viewpoints may lead to a more polarized user base, as individuals become entrenched in their own worldviews without being exposed to opposing arguments.
Thirdly, the suppression of certain content types by algorithms can also have negative consequences for user engagement. For example, if algorithms fail to surface important or nuanced information because it does not generate high levels of engagement, users may feel that the platform is unresponsive to their needs and interests. This could result in decreased usage rates as users seek out alternative platforms where they are more likely to find content that resonates with them. Moreover, this suppression can perpetuate cycles of misinformation, as less prominent but accurate information remains hidden from public view, while sensationalist or false narratives gain undue prominence.
Strategies for Reducing Algorithmic Bias
To mitigate the unintended consequences of algorithmic bias, social media platforms must adopt a multi-faceted approach that addresses both technical and policy challenges. One effective strategy involves diversifying the data sets used to train recommendation algorithms, ensuring that they accurately reflect the diversity of user preferences and interests.
Firstly, incorporating more diverse data sources can help algorithms avoid reinforcing existing biases by providing a broader range of perspectives and content types. For instance, including data from underrepresented communities or alternative viewpoints in training datasets can help ensure that the resulting models are more balanced and inclusive. Additionally, regular audits of algorithmic outputs can identify patterns of bias and guide necessary adjustments to improve fairness.
Secondly, social media platforms should implement transparency measures that allow users to understand how algorithms influence their feed. By providing clear explanations of recommendation criteria and offering options for customization, platforms can empower users to make informed decisions about the content they consume. For example, allowing users to opt-out of certain types of personalized recommendations or adjust settings to prioritize diverse viewpoints can help reduce echo chambers and promote a more balanced information diet.
Thirdly, incorporating human oversight into the algorithmic decision-making process can also play a crucial role in mitigating bias. Human editors or moderators can review flagged content for accuracy and relevance before it is distributed widely, helping to prevent misinformation from gaining traction while ensuring that important but less engaging material still reaches its intended audience. Additionally, involving users in the moderation process through crowdsourced feedback mechanisms can provide valuable insights into potential biases and areas for improvement.
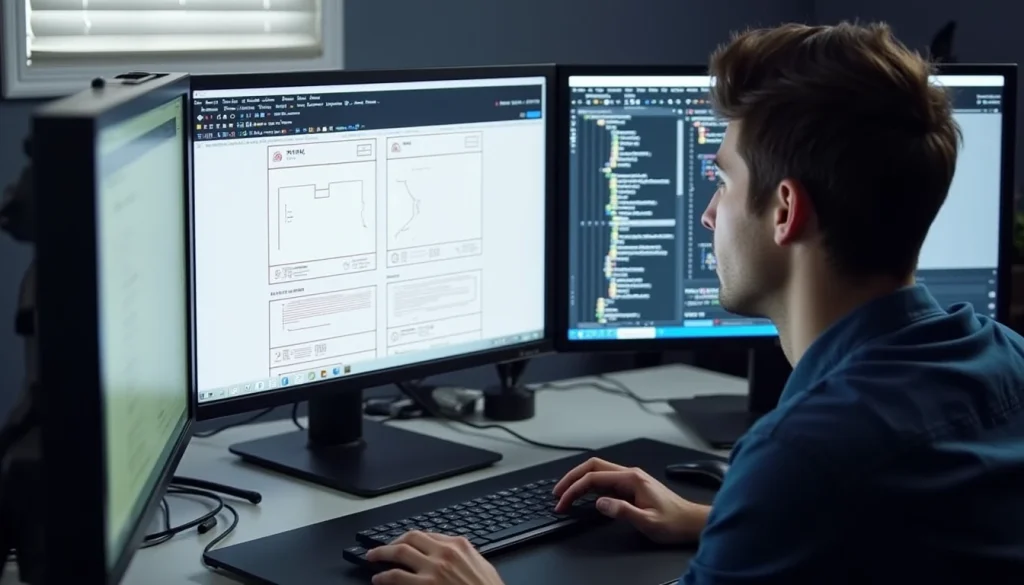
The Role of Regulation in Mitigating Bias
Government regulations and industry standards play a critical role in addressing algorithmic bias within social media platforms. While self-regulation efforts by companies are important, external oversight is necessary to ensure accountability and protect public interests.
Firstly, regulatory bodies should establish clear guidelines for the design and implementation of algorithms used by social media platforms. These guidelines could include requirements for transparency in how algorithms operate, regular audits of algorithmic outputs, and reporting on any identified biases or issues. By setting these standards, regulators can help ensure that companies are held accountable for their actions and provide a framework within which ethical considerations can be integrated into design processes.
Secondly, industry-wide collaboration is essential to develop best practices and share lessons learned across platforms. Through partnerships with academic researchers and civil society organizations, social media companies can access expertise in fields such as data science, sociology, and ethics to inform the development of more equitable algorithms. Additionally, cross-industry initiatives can foster innovation in areas like bias detection tools and content moderation technologies, which could be shared among participating companies.
Thirdly, public education campaigns are crucial for empowering users to recognize and address algorithmic biases when they encounter them on social media platforms. By raising awareness about the mechanisms behind personalization algorithms and providing resources for critical engagement with online content, these campaigns can help individuals navigate information environments more effectively. Furthermore, educating policymakers and regulators on the technical aspects of algorithms can inform regulatory decisions and ensure that new policies are well-informed and effective.
Future Directions in Mitigating Bias
As social media platforms continue to evolve, so too must our approaches to mitigating algorithmic bias. Emerging trends such as artificial intelligence advancements, increased user awareness, and shifting societal values present both challenges and opportunities for improving fairness within these systems.
Firstly, the integration of machine learning techniques with fairness-aware algorithms holds promise for reducing biases in content recommendation systems. These advanced models can be designed specifically to minimize discriminatory outcomes by incorporating fairness constraints during training. By prioritizing equitable treatment across different user groups, these approaches aim to ensure that no demographic is systematically disadvantaged or marginalized within the platform ecosystem.
Secondly, increasing public awareness about algorithmic bias has led to heightened scrutiny of social media practices and growing demands for transparency from users worldwide. This shift in consumer expectations places pressure on companies to adopt more responsible design principles and disclosure policies regarding their algorithms. As a result, platforms may find it beneficial to proactively address concerns related to bias through proactive communication strategies that build trust with their audiences.
Lastly, the changing landscape of societal values also influences how we approach algorithmic fairness. With increasing emphasis placed on diversity, equity, and inclusion, there is renewed interest in developing technologies that promote these ideals online. By aligning technological innovations with broader social goals, researchers and practitioners can work together to create more just and equitable digital spaces where all individuals have equal opportunities for participation and representation.